Acquiring users is important, but what if they don’t stick around? The best Web3 projects prioritize user retention, emphasizing participation metrics such as repeat users, actions taken, and time between actions rather than vanity metrics. Web3 Cohort analysis groups users with similar attributes to uncover engaged segments, highlight retention gaps, and uncover product insights. Let’s dive into how Web3 Cohort Analysis transforms your Web3 retention strategy and turbocharge growth.
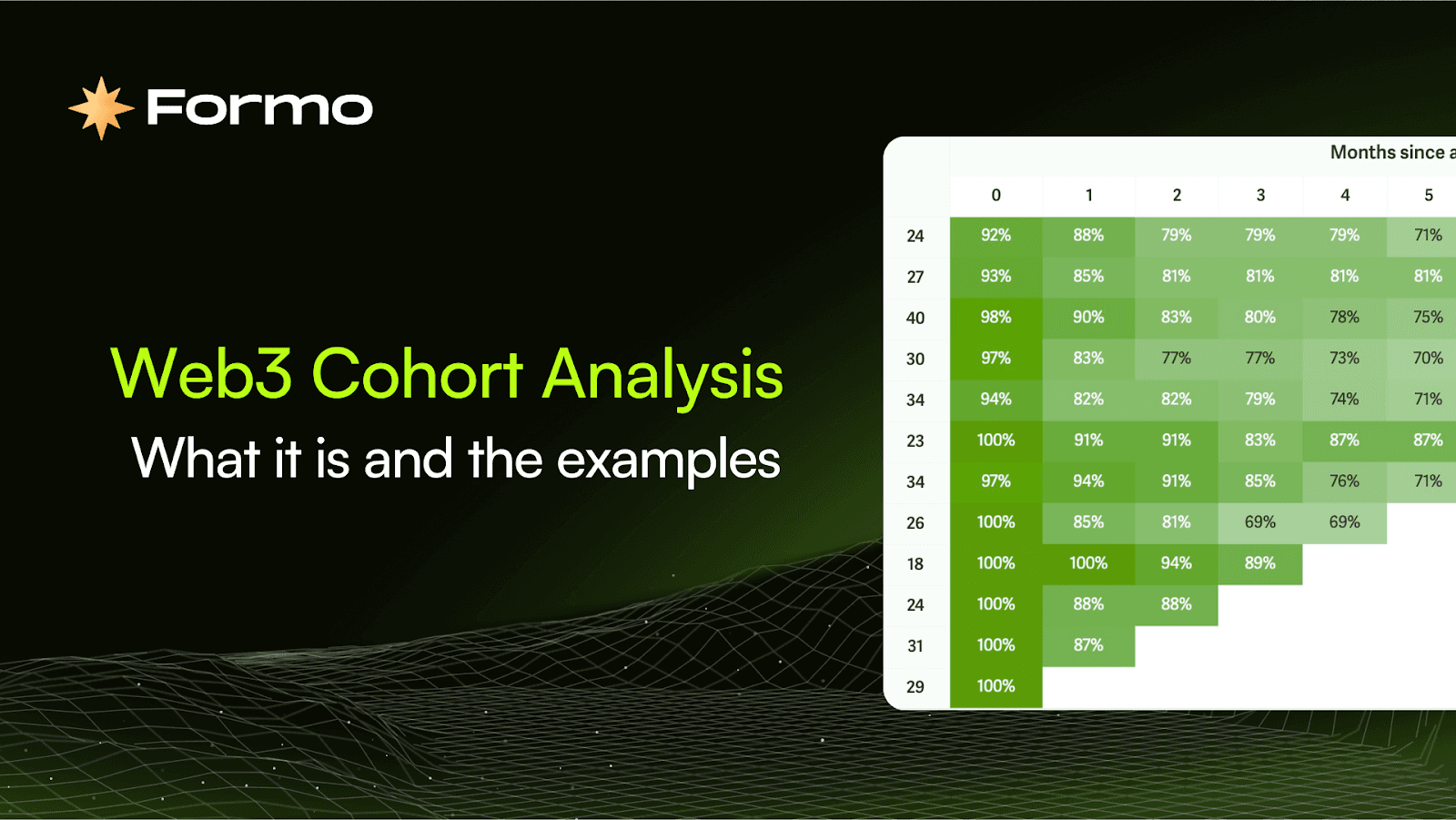
Web3 Cohort Analysis reveals how different user groups interact with a platform over time
What is Web3 Cohort Analysis?
Web3 Cohort Analysis groups users based on dapps used, wallet holdings, or campaigns. This segmentation reveals how different user groups interact with a platform over time - critical for optimizing Web3 user acquisition, retention, and lifetime value (LTV). Cohort analysis is invaluable for understanding user behavior and measuring project success.
Example: Ethereum Cohort Analysis
Track the wallet cohort of users active on Ethereum and observe their retention. Over the next 6 months, we uncover the following insights:
The January cohort included the largest group, with 37M wallets. However, by June, only 4.5M wallets remained active.
Despite the drop-off, 22% of the January cohort remained active by Month 4, marking the highest retention rate.
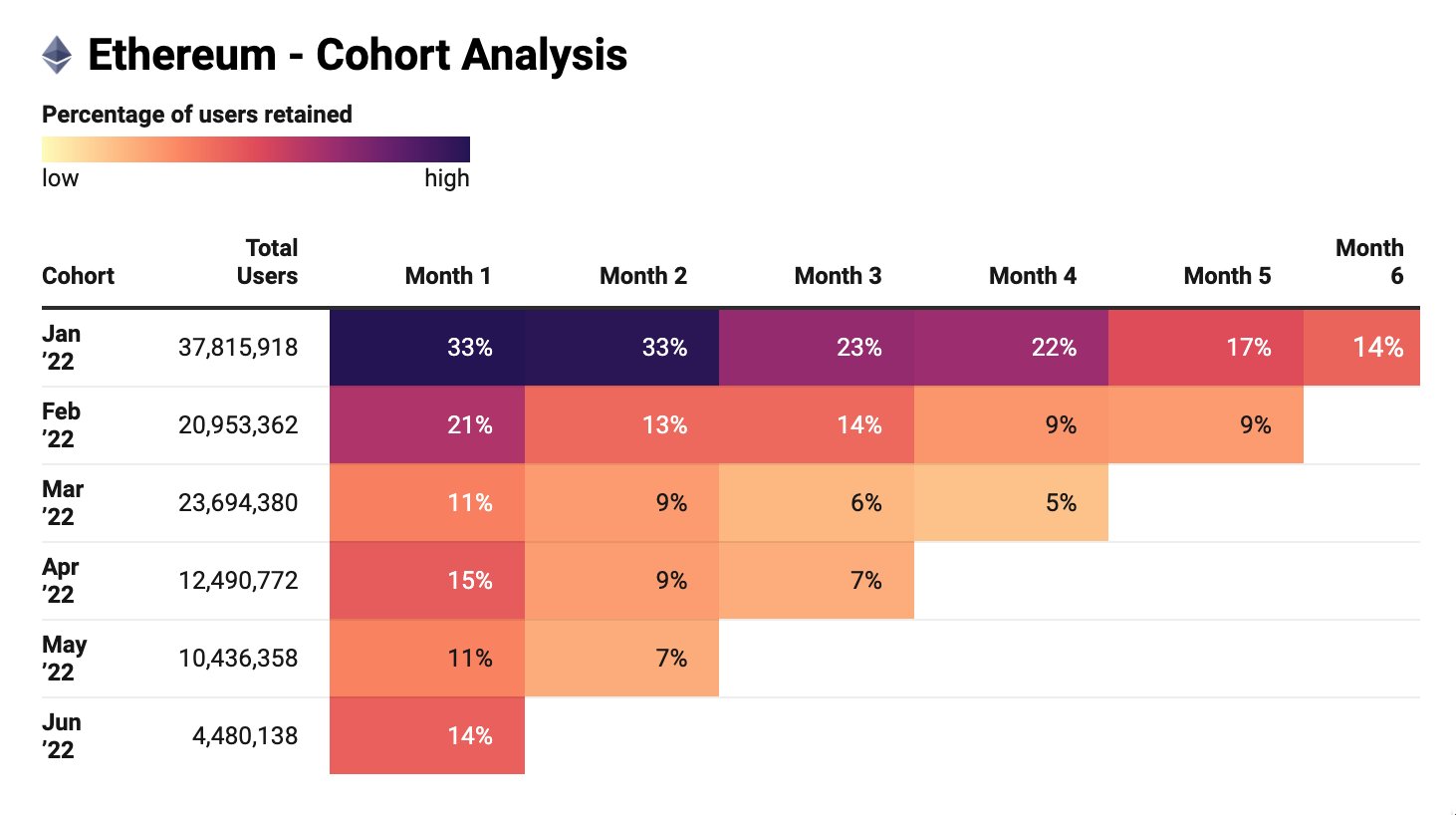
An example of the wallet cohort of users active on Ethereum
Why Web3 Cohort Analysis Matters
User retention remains a major challenge for decentralized applications (dApps). Many projects struggle to sustain meaningful engagement over time, even during market surges. Web3 Cohort analysis provides actionable insights into onchain user behavior, helping you:
Enhance user retention: By analyzing ongoing engagement, Web3 teams identify factors contributing to user drop-off and develop strategies to improve retention rates.
Uncover behavioral insights: Understanding onchain activities empowers more accurate, behavior-driven marketing, such as analyzing how users respond to market changes or platform features.
Improve performance measurement: Evaluating marketing campaigns by cohort performance provides data-driven decisions to inform future initiatives.
Using Web3 analysis, you refine marketing and product strategies, enhance the customer experience, and optimize resource allocation.
Types of Cohort Analysis
Broadly, cohorts are divided into two key categories:
Acquisition cohorts
Acquisition cohorts group users by when they signed up (e.g., day, week, or month). This analysis helps track retention and churn rates, revealing how long users stay engaged from their starting point.
For example, the below retention curve shows a 75% drop-off after Day 1, with further declines to 12% by Day 5 and 11% by Day 10. This pattern often signals that users aren’t quickly accessing the app’s core value, emphasizing the need for a better onboarding experience.
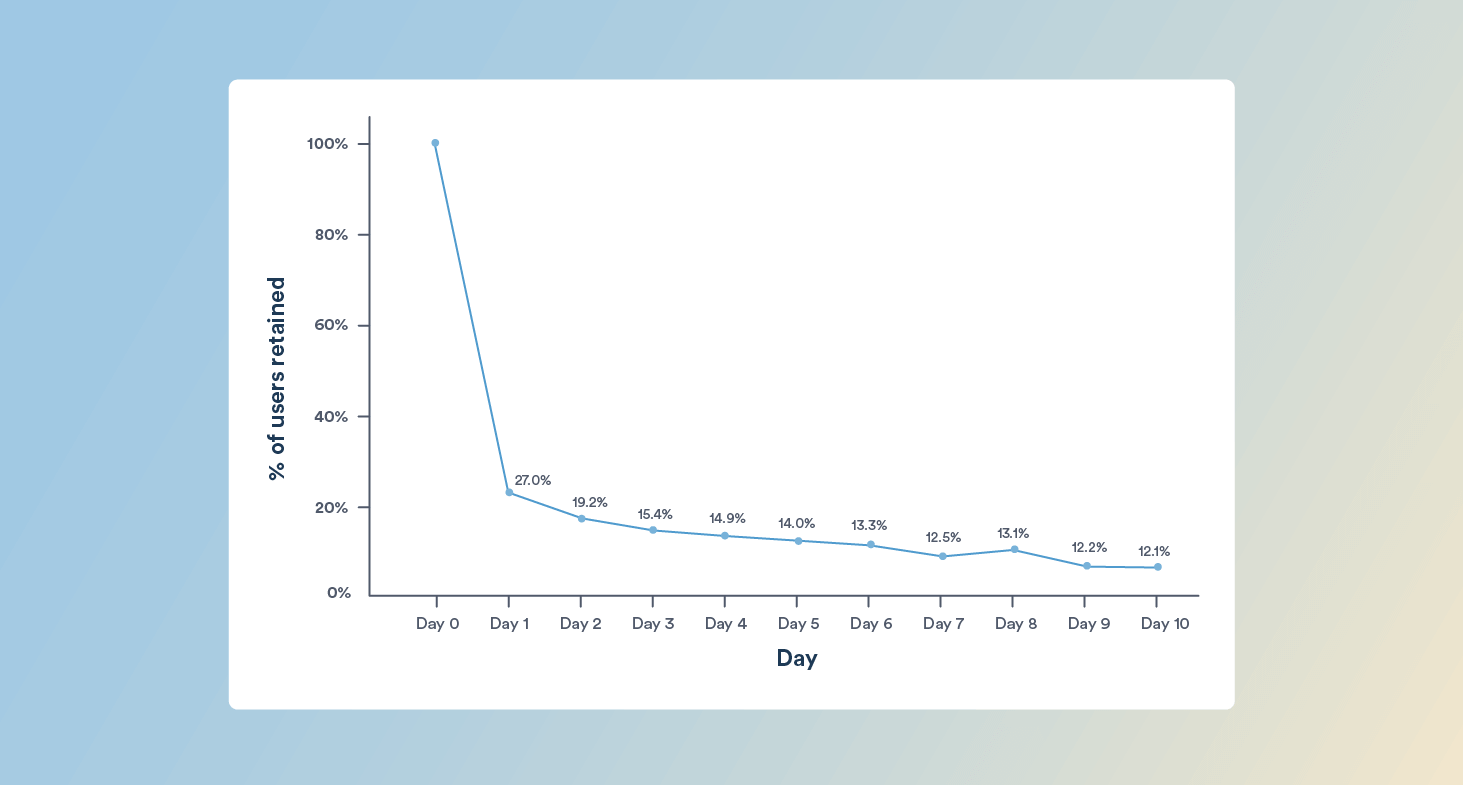
An example of acquisition cohorts
While acquisition cohorts highlight “when” churn happens, we need behavioral cohorts to understand “why”.
Behavioral cohorts
Marketers or analysts categorize behavioral cohorts by specific actions or behaviors, such as app installs, launches, or transactions. For example, analysts examine users who made purchases within three days to understand how their behaviors enhance engagement and retention.
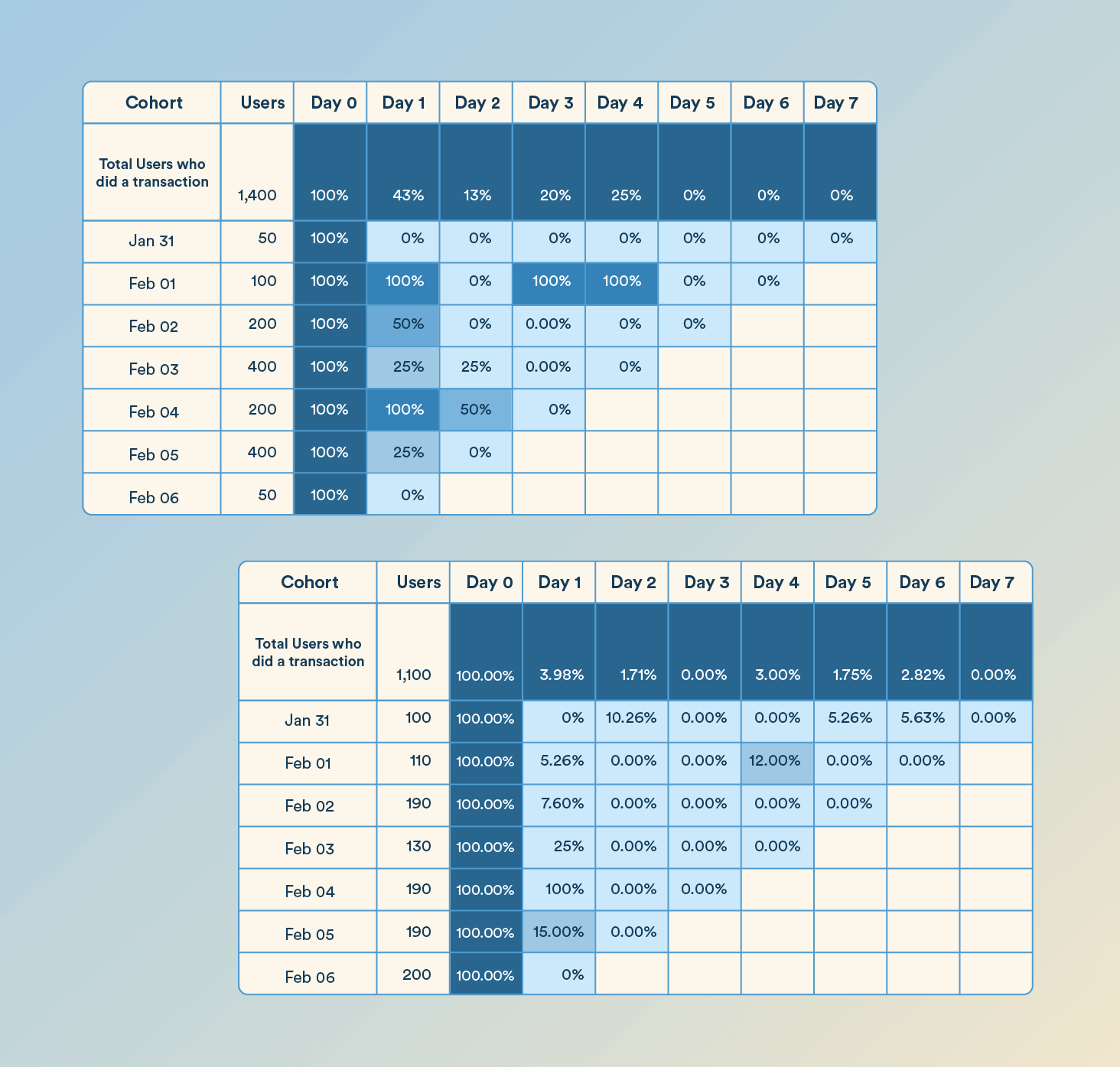
An example of how to track behavioral cohorts
From this group of behaviors, we observe that:
Transaction Cohort: Among 1,400 users, 43% made transactions on Day 1, and by Day 4, retention spiked to 25% after dropping to 13% on Day 2.
Abandoned Cart Cohort: Of 1,110 users, only 3.98% re-engaged on Day 1, declining to 1.71% by Day 2 and minimally improving on Day 4. These users may need targeted offers or reminders soon after cart abandonment.
Comparing these cohorts helps identify key engagement moments and strategies, such as retargeting campaigns or personalized incentives, to reduce churn.
4 steps to conducting a cohort analysis
Here are 4 basic steps to conduct your Web3 cohort analysis
1. Track Churn Timing
Identify when users churn by analyzing acquisition cohorts over time. This helps pinpoint specific drop-off moments and understand the causes.
When you observe the timeline for different user cohorts, you tend to start seeing patterns such as:
When do users stop engaging actively with your product?
Which features did they stop or never start using?
Which types(s) of users cancel their subscriptions and when?
An example of tracking churn timing
2. Identify Sticky Features
Look for major drop-offs in your cohort data and ask what happened on those days. Instead of broad engagement patterns, focus on specific features, user flows, or UX elements that may affect retention.
3. Compare Behavioral Cohorts
It’s often a mix of features and behaviors that drive retention issues. Compare behavioral cohorts to uncover these layers. Cohorts help you track changes in user behavior after signup and understand why. The main goal is to find inflection points and understand if users engage enough with features during onboarding.
4. Iterate and Test
Cohort analysis is not the final goal - it's a step that feeds into A/B testing. The aim is to identify a connection between metrics like churn, upgrades, drop-offs, onboarding completion, or other user engagement indicators and specific variables in your product. Once you establish a relationship between core metrics and a variable, you can modify it and test to see if conditions improve. If there’s no change, discard your initial assumption, return to step three, and continue experimenting.
Web3 Cohort Analysis Example
Let’s start with the retention rate of networks in 2024, based on the user cohort from the beginning of this year.

An example of the retention rate of networks in 2024
Starknet demonstrates crypto’s retention struggles, as its user retention dropped sharply from 18% in March to just 4.3% by later months. Their airdrop campaign’s end likely caused this decline, showing how speculative incentives fail to sustain long-term engagement.
In contrast, networks such as Base and The Open Network (TON) show stronger retention, thanks to their focus on building robust consumer ecosystems and a solid product-market fit.
In this cohort, we see two key areas for improving crypto adoption:
Use Cases: Develop compelling consumer crypto products that generate intrinsic demand and foster engagement.
Distribution: Leverage Web2 channels to introduce Web3 products to non-crypto-native audiences, accelerating the transition from Web2 to Web3.
Formo empowers Web3 brands to establish a strong data foundation and effectively utilize onchain data to drive growth and success. If your Web3 product and marketing teams want to improve their data-driven decision-making and user targeting, contact us to set up and implement a comprehensive cohort analysis strategy.
Web3 Cohort Analysis is a cutting-edge tool to understand Web3 user behavior and improve retention by identifying when and why users drop off. provides the data-driven clarity needed to make informed decisions to enhance customer churn analysis, identify sticky features, and improve your onboarding process.
Follow us on LinkedIn and Twitter, and join our community to learn how Formo empowers builders across web3!